How to Overcome the Challenges of Forecasting New Products
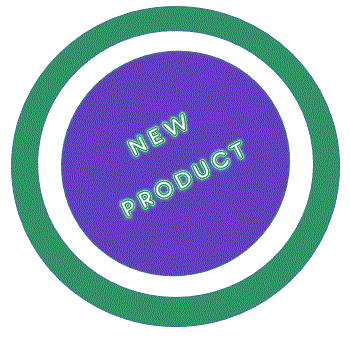
Mark Twain noted that, “It is hard to make predictions, particularly about the future.” However, demand forecasting requires daily predictions to keep the business running smoothly. One of the most difficult areas of forecasting is new product forecasting. There are fewer tools and much less data available for new product forecasting than that which is available when making traditional demand forecasts. However, there are several steps that you can take to improve the accuracy of your new product forecasts.
Understanding the Challenges in Forecasting New Products
Before you can solve the problems presented by new product forecasting, it is essential that you fully understand all of the challenges. The most common challenges in this area are:
- Limited availability of data
- Tight deadlines for forecasts
- Organization bias
- Unknown unknowns
- Low accuracy
Limited availability of data
The starting point for standard demand forecasting is to examine historical data and current sales figures. For most new products neither of these exist. However, the mistake many make in new product forecasting is to assume that there is no data available. However, if the new product is similar to an existing product, you may be able to use the historical data from that product as a guideline in the new product forecast.
Tight deadlines for forecasts
While demand forecasting is almost always done under incredible time pressure, the situation is often worse for new products. The lack of information and the rush to get a product to market make the time pressure especially acute. Increased time pressure often leads to errors in the forecasting process. One of the ways to ameliorate the time pressure for new product forecasts is to have stronger internal communication about the timeline for new products and the realities of new product forecasting.
Organizational bias
Companies create new products to make money. There are often substantial investments made in the research and development of a product. The different stakeholders not only want the product to succeed, but they need the product to succeed. This can result in an organizational bias towards optimistic new product forecasts. Nobody wants to hear that a product will lose money.
Unknown unknowns
The lack of relevant data and tight time pressure are compounded with the fog that surrounds any new product. There are always going to be things that you don’t know. But, there will also be things that you do not even know that you don’t know. These surprises can only be discovered after the product is launched. One way to limit the danger these unknowns pose is to have a slow roll out, or extensive beta testing.
Low accuracy
The notoriously poor accuracy of new product forecasting is the result of the various factors already discussed. The general understanding that new product forecasts are not accurate can also become a self-fulfilling prophecy. Some stakeholders may not take warnings in the forecast seriously, because they believe most new product forecast to be inaccurate. This results in the company not taking the steps to improve the new product introduction (NPI) process that could have made the launch more profitable.
Some industries struggle more than others with new product forecasting. The grocery industry often sees new products fail 70-80% of the time. Other industries with short product lifecycles, such as the mobile phone industry, also are notoriously difficult to accurately forecast.
Effective Methods for Forecasting New Products
There are a variety of different methods for forecasting new products. None of them are perfect. Each method is prone to different biases. The most important consideration when choosing a forecasting method is to also develop a plan to compensate for the deficiencies of the method.
Four effective methods for forecasting new products are:
- Historical review
- Traditional awareness
- Test market
- Before and after retail simulation
Historical review
This method only works if the new product is similar to other products launched into similar markets in the past. This will not work for paradigm shifting products or for new markets.
This method looks to the company’s historical data for guidelines on how the new product will perform. It can also draw upon publicly available data from competitors for their similar products.
However, similar products may have enough differences that the use of historical data can be problematic. Additionally, if the data is too old, it may not reflect the realities of the current marketplace. Using the historical review method does have the advantage of being based on real sales data.
Traditional awareness
This is the method that companies have used for generations when forecasting new products. The first step in this process is to calculate the awareness of the new product in the marketplace. Awareness is calculated based on the planned media campaign.
The rates of product awareness are then fed into a mathematical model that will calculate a cumulative trial rate. Samples of the product are used by customers under real world conditions. The data from these trials can then be used to indicate the repeat purchase curve and the lifecycle of the product. These numbers can then be combined with the exposure numbers to calculate how many of the products will be sold over the first year.
While this method has stood the test of time, there is some concern it does not accurately account for the way media is currently being used. It also requires a lengthy trial period not suitable for all product classes.
Test market
Before a product is rolled out on a large scale, it is introduced to a small geographically discrete segment of the market. Sales and other related data are carefully tracked. After an initial trial period, these numbers are extrapolated for the entire marketplace.
One issue with this method is the length of time it takes to bring the product all the way to market. Many products have too short a life cycle to use this method.
Before and after retail simulation
A carefully selected cross section of likely customers are presented with a realistic simulation of a retail environment. The competitor’s products are displayed as they would be in a store. The sample customers are invited to purchase as they normally would and as they would for their next ten purchases. In the before simulation the new product is absent. The customers are then exposed to advertising or marketing for the new product. They then go back to the simulation. The display is the same as before except, the new product is now there.
This method has the advantage of being faster than using test markets, but may overstate the impact the new product will have.
Once you understand the challenges of forecasting a new product and the tools available to you, you will have a better chance at producing accurate forecasts. When designing your forecast, make sure to account for known biases. Ultimately, like all forecasting, the more times you do it, the more skilled you will become.
Written by Jamsheed Iqbal
Topics:
Leave a Reply Cancel reply
Recent Posts
-
Best Practices for Demand Sensing, Demand Shaping, & Influencing Demand
Despite vast improvements in the tools and techniques available today for demand sensing and demand shaping, a lack of forecast accuracy still prevents many businesses from optimally managing production, distribution, inventories, and sales to meet shifts in demand. This is often because:
October 12, 2021 Read more -
How to Effectively (and Accurately) Forecast and Plan for New Product Demand
Many businesses, large and small, have a huge source of great ideas that can help them improve, innovate, and grow, and yet so many of these companies never think of using this amazing corporate asset. What is this highly valuable asset? Its own people.
September 28, 2021 Read more -
How to Measure your Demand Forecasting Accuracy
Many businesses, large and small, have a huge source of great ideas that can help them improve, innovate, and grow, and yet so many of these companies never think of using this amazing corporate asset. What is this highly valuable asset? Its own people.
July 13, 2021 Read more
Subscribe to email updates from Causometrix!
Stay up-to-date with Causometrix
custom essay writing service
Writing is conventional method to verbal communication.
The good writing involves good idea, focusing on ideas clearly, argument or the thesis statement of the topic based on which the article is prepared.
I found your site as a perfect platform to get good contents to read. I have noticed these entire things in your blog.
I need to get good skill and knowledge as I am a writer at custom essay writing service. Thank you for the helpful posts.